- Python Visualization Examples
- Python Visual Studio Tutorial
- Python Visual Studio Vs Code
- Python Visualization
- One of the coolest code editors available to programmers, Visual Studio Code, is an open-source, extensible, light-weight editor available on all platforms. It’s these qualities that make Visual Studio Code from Microsoft very popular, and a great platform for Python development.
- Create Python visuals in Power BI Desktop Select the Python visual icon in the Visualizations pane. In the Enable script visuals dialog box that appears, select Enable. When you add a Python visual to a report, Power BI. A placeholder Python visual image appears on the report canvas.
- Publishing Power BI reports with Python visuals. You can add a Python visual to your Power BI report by selecting the Python visual icon from the Visualizations pane in the Power BI Desktop, which adds a placeholder Python script to the report. Adding the fields from your dataset to the Values pane of the Python visual, automatically adds the.
With Power BI Desktop, you can use Python to visualize your data.
VPython makes it easy to create navigable 3D displays and animations, even for those with limited programming experience. Because it is based on Python, it also has much to offer for experienced programmers and researchers. Click the 'Run' button above to see a 3D animation.
Prerequisites
Work through the Run Python scripts in Power BI Desktop tutorial using the following Python script:
The Run Python scripts in Power BI Desktop article shows you how to install Python on your local machine and enable it for Python scripting in Power BI Desktop. This tutorial uses data from the above script to illustrate creating Python visuals.
Create Python visuals in Power BI Desktop
Select the Python visual icon in the Visualizations pane.
In the Enable script visuals dialog box that appears, select Enable.
When you add a Python visual to a report, Power BI Desktop takes the following actions:
A placeholder Python visual image appears on the report canvas.
The Python script editor appears along the bottom of the center pane.
Next, drag the Age, Children, Fname, Gender, Pets, State, and Weight fields to the Values section where it says Add data fields here.
Your Python script can only use fields added to the Values section. You can add or remove fields from the Values section while working on your Python script. Power BI Desktop automatically detects field changes.
Note
The default aggregation type for Python visuals is do not summarize.
Now you can use the data you selected to create a plot.
As you select or remove fields, supporting code in the Python script editor is automatically generated or removed.
Based on your selections, the Python script editor generates the following binding code.
- The editor created a dataset dataframe, with the fields you added.
- The default aggregation is: do not summarize.
- Similar to table visuals, fields are grouped and duplicate rows appear only once.
Tip
In certain cases, you might not want automatic grouping to occur, or you'll want all rows to appear, including duplicates. If so, you can add an index field to your dataset that causes all rows to be considered unique and which prevents grouping.
You can access columns in the dataset using their respective names. For example, you can code
dataset['Age']
in your Python script to access the age field.With the dataframe automatically generated by the fields you selected, you're ready to write a Python script that results in plotting to the Python default device. When the script is complete, select Run from the Python script editor title bar.
Power BI Desktop replots the visual if any of the following events occur:
- When you select Run from the Python script editor title bar
- Whenever a data change occurs, due to data refresh, filtering, or highlighting
When you run a Python script that results in an error, the Python visual isn't plotted and a canvas error message appears. For error details, select See details from the message.
To get a larger view of the visualizations, you can minimize the Python script editor.
Ok, let's create some visuals.

Create a scatter plot
Let's create a scatter plot to see if there's a correlation between age and weight.
Under Paste or type your script code here, enter this code:
Your Python script editor pane should now look like this:
The matplotlib library is imported to plot and create our visuals.
When you select the Run script button, the following scatter plot generates in the placeholder Python visual image.
Create a line plot with multiple columns
Let's create a line plot for each person showing their number of children and pets. Remove or comment the code under Paste or type your script code here and enter this Python code:
When you select the Run script button, the following line plot with multiple columns generates.
Create a bar plot
Let's create a bar plot for each person's age. Remove or comment the code under Paste or type your script code here and enter this Python code:
Python Visualization Examples
When you select the Run script button, the following bar plot generates:
Security
Important
Python scripts security: Python visuals are created from Python scripts, which could contain code with security or privacy risks. When attempting to view or interact with an Python visual for the first time, a user is presented with a security warning message. Only enable Python visuals if you trust the author and source, or after you review and understand the Python script.
More information about plotting with Matplotlib, Pandas, and Python
This tutorial is designed to help you get started creating visuals with Python in Power BI Desktop. It barely scratches the surface about the many options and capabilities for creating visual reports using Python, Pandas, and the Matplotlib library. There's a lot more information out there, and here are a few links to get you started.
- Documentation at the Matplotlib website.
Known limitations
Python visuals in Power BI Desktop have a few limitations:
- Data size limitations. Data used by the Python visual for plotting is limited to 150,000 rows. If more than 150,000 rows are selected, only the top 150,000 rows are used and a message is displayed on the image. Additionally, the input data has a limit of 250 MB.
- If the input dataset of a Python Visual has a column that contains a string value longer than 32766 characters, that value is truncated.
- Resolution. All Python visuals are displayed at 72 DPI.
- Calculation time limitation. If a Python visual calculation exceeds five minutes the execution times out which results in an error.
- Relationships. As with other Power BI Desktop visuals, if data fields from different tables with no defined relationship between them are selected, an error occurs.
- Python visuals are refreshed upon data updates, filtering, and highlighting. However, the image itself isn't interactive and can't be the source of cross-filtering.
- Python visuals respond to highlighting other visuals, but you can't click on elements in the Python visual to cross filter other elements.
- Only plots that are plotted to the Python default display device are displayed correctly on the canvas. Avoid explicitly using a different Python display device.
- Python visuals do not support renaming input columns. Columns will be referred to by their original name during script execution.
Next steps
Take a look at the following additional information about Python in Power BI.
The addition of Python integration in Power BI is one of the greatest things that Microsoft could have done. This gives the user the ability to utilize amazing visual libraries such as Seaborn. Beyond visualization, you can also utilize some of the machine learning packages.
If you want to improve your data visualization skill, check out Use Power BI for Data Science.
Also, check out our blog on how to Do Machine Learning in Power BI.
The first thing you will need is the latest version of Power BI, this will have the Python integration in the preview features, you can enable this and then restart. Just go to your options and enable the feature.
Use the Python Script Editor and Visual Tile
1. Click the Python visual within the Visualization panel. This will open the script editor window at the bottom of the page.
When this icon is clicked, the script editor opens with a visual tile that is empty. I am using Google Analytics data to make my dataset.
2. You will need to add your values to build your dataset by selecting the fields which will fill your values section to create the data you will use in a visual. Once you have the dataset built you will see it as a pandas data frame which can be thought of as a table.
3. Now you will need to import some libraries to ensure you can create the visuals. use the following script to import Matplotlib and Seaborn. We are using abbreviations of plt for and seaborn to make our code more efficient.
Violin Plot with Seaborn Libary
This imports the visuals library that will allow you to create some cool visuals. Now let’s build some plots aka visuals. Since we have the first part of the code, let’s add to the code to get the visuals we want. The first visuals we will create is a violin plot. We are going to use the values that we added in which creates our dataset.
Paste the Code:
Using this code will produce a violin plot which will show you where the concentration of session duration is on the y-axis by the device.
Python Visual Studio Tutorial
We can see from the desktop has a higher amount of outliers with higher session durations than any other device. We can evaluate the gender by simply changing the x-axis to gender.
Create a Joint Plot
The next plot is going to joint plot. This works off the same backbone of code that you’ve already built using the violinplot. The only difference is that you are going to exchange sns.violinplot for sns.jointplot. However, a joint plot is a modified scatter plot so your X and Y variables will need numerical. For this example, I wanted to know if there was a correlation between session duration and goal completions. you can see from my code below that I exchange that I used Session Duration as my x-axis and Goal Completions my y-axis
Paste the Code:
This plot doesn’t award me a lot of insights into my goal completions. We can change the x variable into page views to see if we get a better correlation score which is listed in the top right.
Create a Heatmap Correlation Plot
Now let’s create some correlation based on our dataset. This is a fun way to do some exploratory data analysis. We can do this by using a heat map which is a very powerful visual. Since we are going to be evaluating the whole dataset, we don’t need to define the x and y-axis. We can get a correlation score for every variable.
Paste the Code:
The code takes in the whole dataset, I added a color gradient called ‘coolwarm’ however you can explore tons of color gradient. This was added with the cmap parameter. You can check out the Seaborn website to see other colors scales you can use.
We can further customize this visual by added no parameters such as labels and line width. This will make the heat map more actionable by displaying the correlations.
Paste the Code:
Create a Seaborn Pair Plot
The last plot we are going to create is the easiest. Its called a pair plot which is essentially an aggregation of all your dataset correlation similar in separate graphs. It gives you a quick and simple look at your correlations for deeper exploration.
Python Visual Studio Vs Code
Paste the Code:
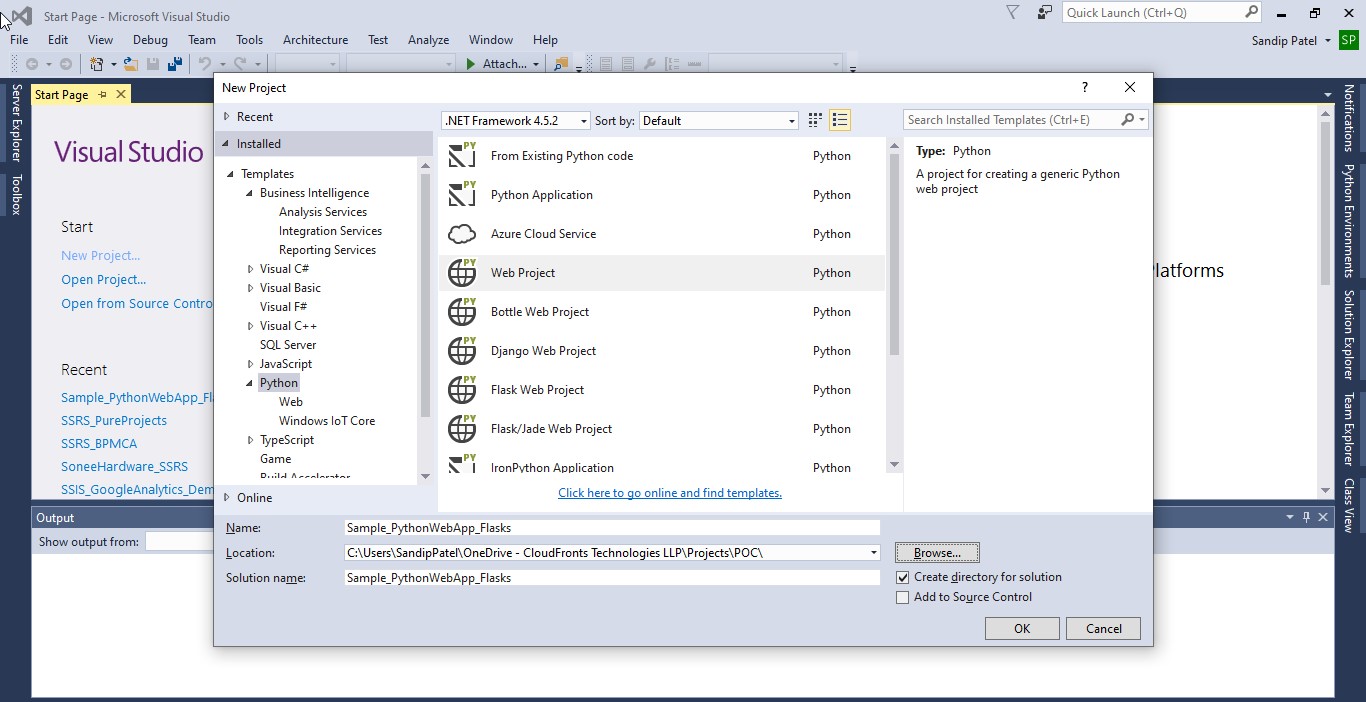
Python Visualization
Lastly, you can display these visuals as a report in any fashion that you like.
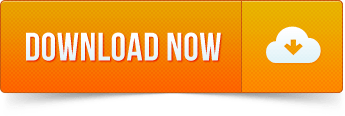
Comments are closed.